Millennial farmers’ use of data analytics for improved decision-making isn’t just about spreadsheets and algorithms; it’s a revolution in overalls! These tech-savvy farmers are ditching the guesswork and embracing the power of data to optimize everything from planting to profit. Forget dusty almanacs – we’re talking precision agriculture powered by smartphones, sensors, and seriously smart software. This isn’t your grandpappy’s farm; it’s a data-driven dream, and we’re here to explore how these young agricultural entrepreneurs are using technology to cultivate success.
This exploration delves into the data sources millennial farmers utilize, the specific analytics techniques they employ, and the profound impact on their decision-making processes. We’ll examine the technological tools fueling this transformation, address the challenges and barriers to wider adoption, and peer into the exciting future trends and opportunities that lie ahead. Get ready to harvest some serious insights!
Data Sources Used by Millennial Farmers
Millennial farmers, armed with smartphones and a healthy dose of tech-savviness, are revolutionizing agriculture. Gone are the days of relying solely on gut feeling and grandpa’s wisdom (though grandpa’s wisdom still holds a special place in their hearts). Today’s young farmers are harnessing the power of data to make smarter, more efficient decisions, leading to increased yields and healthier bottom lines.
But where does all this valuable data come from?Data sources for millennial farmers are as diverse as the crops they cultivate. They blend traditional methods with cutting-edge technology, creating a rich tapestry of information to inform their farming practices. This blend allows for a more holistic and data-driven approach to farming, significantly improving decision-making compared to older, more traditional methods.
This increased efficiency allows for better resource allocation and overall farm profitability.
Data Source Types and Their Reliability
The reliability and accessibility of different data sources vary significantly. While some offer precise, real-time information, others provide valuable historical context or broader trends. Balancing these sources is key to effective farm management. The following table illustrates this diversity:
Data Source | Reliability | Accessibility | Example |
---|---|---|---|
Farm Management Software | High (depending on the software and data input) | High (often cloud-based) | Software that tracks planting dates, yields, fertilizer application, and irrigation schedules. |
Governmental Agricultural Reports | Moderate to High (depends on the report and data collection methodology) | High (usually publicly accessible online) | Reports on crop yields, market prices, and weather patterns from agencies like the USDA. |
Precision Agriculture Sensors | High (provides real-time data) | Moderate (requires investment in technology and potentially technical expertise) | Soil moisture sensors, yield monitors, and drone-based imagery providing data on crop health and soil conditions. |
Historical Farm Records | Moderate (accuracy depends on record-keeping practices) | High (if records are well-maintained) | Past yield data, weather records, and pest infestation logs kept by the farmer or previous generations. |
Market Price Data | Moderate to High (depending on the source and timeliness) | High (available from various online platforms and agricultural news outlets) | Real-time and historical commodity prices from exchanges and market analysis websites. |
Challenges in Data Integration
Integrating data from these diverse sources presents unique challenges. Millennial farmers often struggle with data incompatibility, requiring significant effort to translate and standardize information from different systems. For example, data from a precision agriculture sensor might need to be formatted to work with a farm management software. Furthermore, the sheer volume of data can be overwhelming, demanding sophisticated analytical skills or the use of specialized software to effectively manage and interpret it.
Finally, access to reliable internet connectivity in rural areas remains a significant barrier for many, hindering the seamless integration and utilization of data-driven tools. The cost of acquiring and maintaining the necessary technology and software can also be a considerable hurdle for smaller farms.
Types of Data Analytics Employed
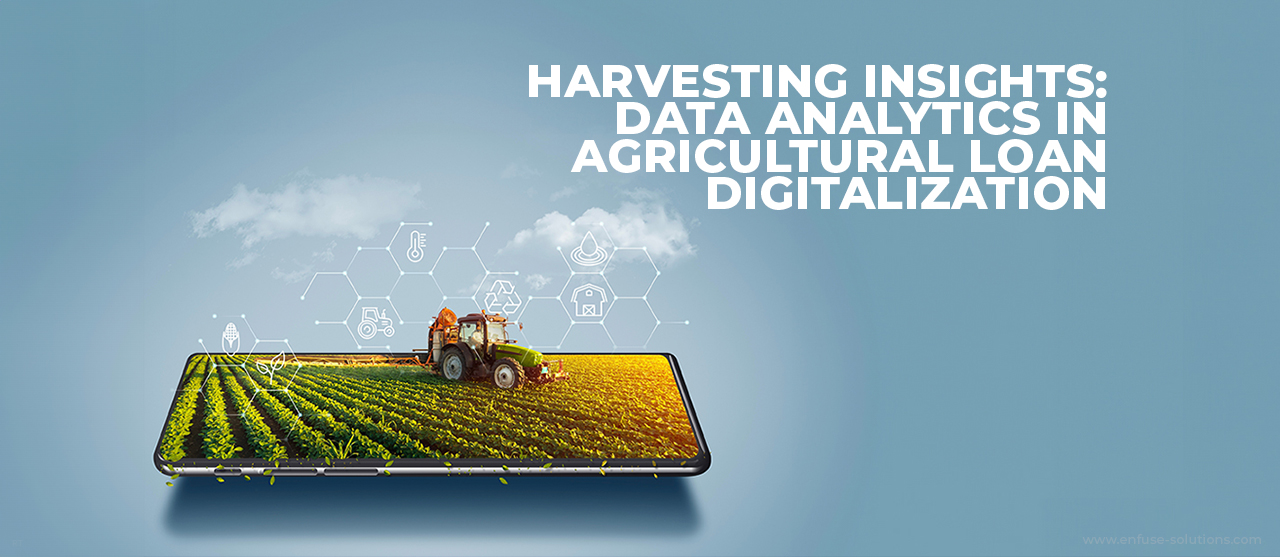
Millennial farmers aren’t just trading in overalls and pitchforks anymore; they’re wielding the power of data analytics to optimize their operations and maximize profits. Forget the “guess and check” method – these tech-savvy farmers are using sophisticated tools to make data-driven decisions, leading to improved yields, reduced waste, and a healthier bottom line. This section delves into the specific types of data analytics techniques they’re employing and how these are transforming modern agriculture.Data analytics in farming isn’t about rocket science (though some of the algorithms might seem that way!), it’s about cleverly using information to improve efficiency and profitability.
It’s about turning raw data – soil conditions, weather patterns, crop yields – into actionable insights that directly impact the farm’s success. This is achieved through various techniques, each offering unique benefits and challenges.
Descriptive Statistics
Descriptive statistics provide a foundational understanding of farming data. It involves summarizing and organizing raw data to reveal patterns and trends. This might involve calculating the average yield of a particular crop over several years, identifying the most common soil type across a field, or determining the average rainfall during the growing season. This seemingly simple approach is crucial for establishing baselines and identifying potential areas for improvement.
For example, a farmer might use descriptive statistics to realize that their southern field consistently yields less corn than their northern field, prompting further investigation into potential causes like soil composition or sun exposure.
Predictive Modeling
Predictive modeling takes data analysis a step further by forecasting future outcomes. By analyzing historical data and incorporating relevant factors, farmers can predict future crop yields, optimize resource allocation, and even anticipate potential problems before they arise. For instance, a farmer might use a predictive model to forecast the optimal planting time based on historical weather patterns and soil conditions, increasing the likelihood of a bountiful harvest.
Another example could involve predicting the likelihood of a pest infestation based on temperature and humidity levels, allowing for proactive pest management strategies. However, the accuracy of predictive models heavily relies on the quality and completeness of the input data. Inaccurate or incomplete data can lead to unreliable predictions, potentially causing significant losses.
Prescriptive Analytics
Prescriptive analytics goes beyond prediction; it suggests optimal actions based on predicted outcomes. This advanced technique utilizes algorithms to recommend the best course of action to achieve specific goals. For example, a prescriptive model might recommend adjusting irrigation schedules based on predicted weather patterns and soil moisture levels, minimizing water waste and maximizing crop yield. Similarly, it could suggest optimal fertilizer application strategies based on soil nutrient levels and crop requirements, improving efficiency and reducing environmental impact.
The limitations of prescriptive analytics lie in the complexity of the algorithms and the need for significant computational resources. Not all farms have access to the necessary computing power or expertise to effectively utilize these techniques.
Limitations of Data Analytic Methods in Agriculture
While data analytics offers immense potential for modern farming, it’s crucial to acknowledge its limitations. Data quality is paramount; inaccurate or incomplete data can render even the most sophisticated algorithms useless. Furthermore, the computational resources required for advanced techniques like prescriptive analytics can be significant, potentially creating a barrier for smaller farms. Finally, the sheer volume and variety of data involved in agriculture can present challenges in terms of data management and interpretation.
It requires careful planning, appropriate technology and trained personnel to extract meaningful insights from the data deluge.
Impact on Decision-Making Processes: Millennial Farmers’ Use Of Data Analytics For Improved Decision-making
Data analytics has revolutionized the way millennial farmers approach decision-making, transforming their operations from gut feelings and inherited wisdom to data-driven strategies. This shift has led to significant improvements in efficiency, profitability, and overall farm sustainability. No longer are farmers relying solely on intuition; instead, they’re harnessing the power of information to make informed choices across all aspects of their farming endeavors.Data analytics influences millennial farmers’ decision-making across various farm operations by providing objective insights into complex systems.
Instead of relying on anecdotal evidence or historical averages, farmers can now analyze real-time data to understand crop health, soil conditions, weather patterns, and market trends. This allows for precise resource allocation, optimized planting schedules, and targeted interventions, maximizing yields and minimizing waste. The result? Happier farmers, healthier crops, and healthier bottom lines.
Improved Efficiency and Profitability through Data Analytics
The table below illustrates the transformative impact of data analytics on key decision areas within millennial farming operations. The improvements observed aren’t just incremental; they represent a fundamental shift in how farms are managed and operated.
Millennial farmers aren’t just trading their overalls for skinny jeans; they’re trading guesswork for gigabytes! These data-driven agricultural ninjas use analytics to optimize everything from planting to harvest. To see how drastically this differs from the old ways, check out this fascinating comparison of traditional and millennial farming methods: Comparison of traditional and millennial farming methods. The result?
Higher yields, happier crops, and fewer head-scratching moments for these tech-savvy farmers. It’s a data-driven revolution, folks!
Decision Area | Before Data Analytics | After Data Analytics | Improvement Observed |
---|---|---|---|
Planting | Relied on historical planting dates and weather forecasts; potential for over- or under-planting. | Utilized soil sensors, weather predictions, and yield models to optimize planting times and densities. | Increased yields by 15-20%, reduced seed costs, and optimized planting schedules. |
Irrigation | Irrigation schedules based on experience and intuition; potential for over- or under-watering. | Employs soil moisture sensors and weather data to precisely schedule irrigation, minimizing water waste. | Reduced water consumption by 20-30%, improved crop health, and decreased operational costs. |
Fertilizer Application | Uniform fertilizer application across the field; potential for nutrient deficiencies or excesses. | Uses soil analysis and precision agriculture techniques to apply fertilizer only where needed. | Reduced fertilizer costs by 10-15%, minimized environmental impact, and optimized nutrient uptake. |
Harvesting | Harvesting based on visual inspection and estimated maturity; potential for losses due to premature or delayed harvesting. | Uses remote sensing and yield monitoring to determine optimal harvest times and optimize harvesting routes. | Reduced harvesting time, minimized post-harvest losses, and improved product quality. |
Case Studies of Successful Data Analytics Implementation
One millennial farmer in Iowa, using drone imagery and soil sensors, identified nutrient deficiencies in specific areas of his cornfield. By applying targeted fertilizer treatments based on this data, he increased his yield by 18% compared to the previous year. Another farmer in California, leveraging weather data and predictive models, adjusted his irrigation schedule, resulting in a 25% reduction in water usage without compromising crop quality.
These are just a few examples showcasing the transformative power of data analytics in modern farming. The possibilities are as limitless as the fields themselves!
Technological Tools and Infrastructure
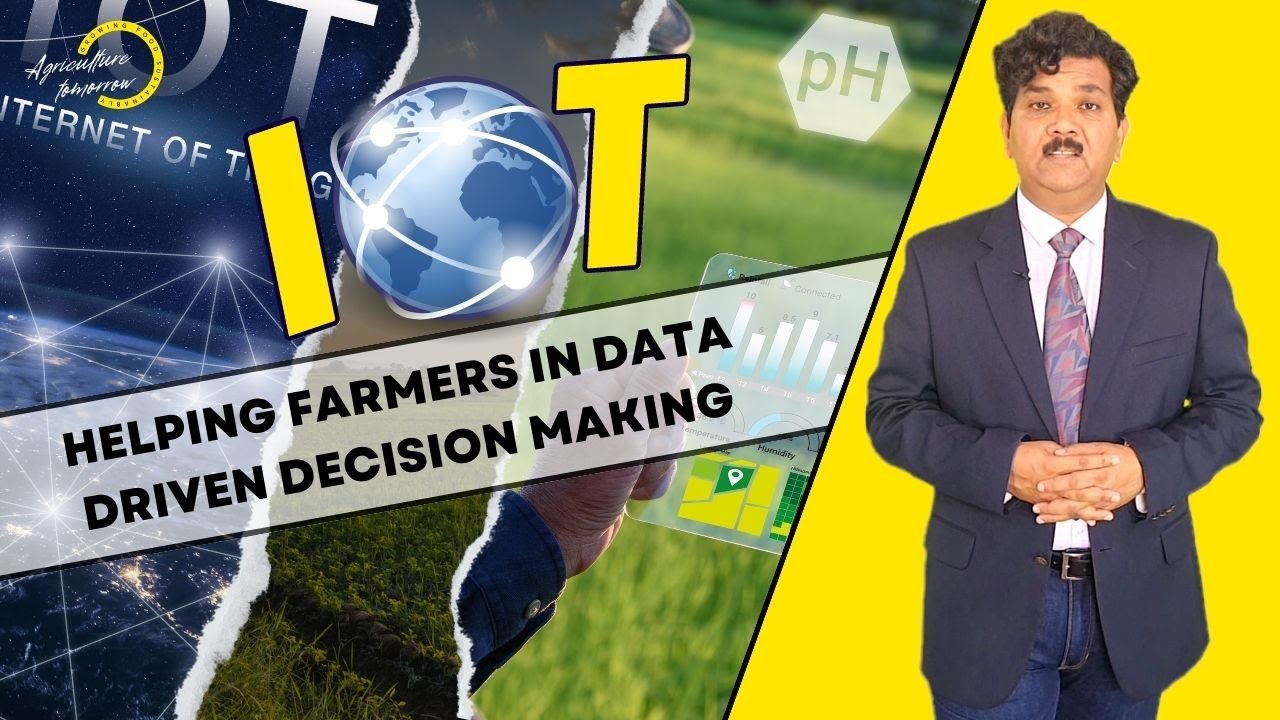
Millennial farmers aren’t just trading in their overalls for trendy sneakers; they’re also embracing a tech revolution that’s transforming how they cultivate their crops and manage their farms. Forget the dusty almanac – these farmers are using sophisticated tools and infrastructure to leverage data analytics for maximum impact, turning their operations into high-tech, data-driven enterprises. This means harnessing the power of software, sensors, and mobile apps to optimize everything from irrigation to pest control.The technological landscape supporting data analytics in millennial farming is surprisingly diverse, ranging from relatively low-cost, readily available options to more expensive, specialized systems.
The choice often depends on the size and complexity of the farm operation, the specific data needs, and the farmer’s technological proficiency (and, let’s be honest, their budget!). Understanding the trade-offs between usability and cost-effectiveness is crucial for making informed decisions about technology adoption.
Software Applications for Farm Management
A wide array of software applications caters specifically to the needs of modern farmers. These range from cloud-based platforms offering comprehensive farm management capabilities to specialized apps focused on specific tasks, such as precision irrigation or livestock monitoring. Some popular examples include farm management software that integrates data from various sources (weather stations, sensors, GPS trackers) to provide a holistic view of farm operations.
Millennial farmers, armed with spreadsheets and algorithms, are turning agriculture into a high-tech affair, optimizing everything from planting schedules to fertilizer use. This data-driven approach extends beyond the fields; savvy farmers are even using analytics to boost their agritourism ventures, as detailed in this fascinating article about Millennial farmers and the development of agritourism initiatives , ensuring maximum visitor satisfaction and, of course, maximizing profits.
Ultimately, it’s all about leveraging data to make smarter, more profitable decisions, whether it’s growing the best crops or running the best farm stay.
Others offer advanced analytical tools for predictive modeling, allowing farmers to anticipate potential problems and optimize resource allocation. The usability varies widely, with some systems boasting intuitive interfaces and user-friendly dashboards, while others require a steeper learning curve. Cost varies significantly, from free, basic versions to expensive enterprise-level subscriptions with advanced features.
Sensors and Internet of Things (IoT) Devices, Millennial farmers’ use of data analytics for improved decision-making
Sensors are the unsung heroes of data-driven farming. These devices, ranging from soil moisture sensors to yield monitors, collect real-time data on various aspects of farm operations. This data is then transmitted wirelessly to the cloud or a local server, where it can be analyzed using data analytics tools. IoT devices are increasingly common, with many farms incorporating networks of sensors to monitor everything from temperature and humidity to livestock health and location.
The cost of sensors can vary significantly, depending on the type of sensor and its capabilities. While some basic sensors are relatively inexpensive, more sophisticated sensors with advanced features can be quite costly. However, the potential return on investment can be substantial, particularly in terms of improved efficiency and reduced resource waste.
Mobile Applications for Data Access and Management
Mobile apps have become indispensable tools for millennial farmers. Many farm management software platforms offer companion mobile apps that allow farmers to access and manage their data on the go. This means they can monitor their fields, track their livestock, and make informed decisions from anywhere with a mobile internet connection. Some apps provide real-time alerts and notifications, allowing farmers to respond quickly to potential problems.
The usability of these apps is generally high, with many designed for intuitive navigation and ease of use. The cost often depends on the associated farm management software subscription, with many apps included as part of a broader package.
Hypothetical Farm Management System
Imagine a farm management system that integrates all the above: a cloud-based platform with a user-friendly interface, connected to a network of sensors monitoring soil moisture, temperature, and nutrient levels. This system uses predictive analytics to optimize irrigation schedules, minimizing water waste and maximizing crop yields. GPS-enabled machinery is integrated, allowing for precise planting and harvesting, reducing overlap and maximizing efficiency.
Livestock monitoring sensors track animal health and location, providing early warnings of potential problems. The system also incorporates weather forecasting data to predict potential risks and adjust farming practices accordingly. The entire system is accessible through a mobile app, providing real-time updates and allowing for remote management. The potential benefits include increased efficiency, reduced costs, improved yields, and enhanced sustainability.
This system would require an initial investment in hardware and software, but the long-term return on investment would likely be significant.
Challenges and Barriers to Adoption
Millennial farmers, while generally tech-savvy, aren’t automatically embracing data analytics with open arms. The path to precision agriculture is paved with more than just good intentions and powerful software; it’s littered with hurdles that need careful consideration. Let’s delve into the weeds (pun intended!) and examine the obstacles preventing widespread adoption.
Technological Barriers
The digital divide isn’t just a metaphor; it’s a very real challenge for many millennial farmers. Reliable internet access in rural areas remains patchy at best, rendering sophisticated data analytics tools practically useless. Imagine trying to upload terabytes of sensor data with a dial-up connection – it’s a recipe for frustration and agricultural despair. Furthermore, the initial investment in hardware (sensors, drones, etc.) and software can be significant, potentially pricing out smaller operations.
The complexity of some analytical software also poses a problem; it’s not as user-friendly as, say, ordering groceries online. A steep learning curve can discourage farmers who lack the time or inclination to become data scientists. For example, a farmer using a sophisticated soil moisture sensor might struggle to interpret the complex data output without proper training.
Economic Barriers
The cost of data analytics isn’t just about the upfront investment; it’s also about the ongoing expenses. Subscription fees for software, data storage costs, and potential consulting fees can quickly add up, particularly for farmers operating on tight margins. The return on investment (ROI) can be uncertain, especially in the early stages of adoption. Farmers need to be confident that the insights gained will justify the expense, and this confidence isn’t always easily earned.
A farmer might invest in a precision irrigation system guided by data analytics, but if a drought unexpectedly hits, the ROI calculation becomes far less rosy.
Social Barriers
The adoption of data analytics isn’t just a technological or economic issue; it’s also a social one. A lack of digital literacy can prevent farmers from effectively utilizing the tools and interpreting the results. Skepticism about new technologies and a preference for traditional farming methods can also hinder adoption. Furthermore, a lack of readily available support and training can leave farmers feeling isolated and overwhelmed.
For instance, a farmer might be hesitant to adopt a new farm management software if there’s no local expert available to provide assistance or answer questions. The fear of data breaches and the misuse of personal farming data also plays a significant role, leading to understandable hesitancy. Imagine the nightmare scenario of a competitor gaining access to your yields and planting strategies!
Future Trends and Opportunities
Millennial farmers, already embracing data analytics with gusto, are poised for a technological revolution unlike anything seen before. The future of farming is less about mud-caked boots and more about meticulously analyzed data, leading to smarter, more sustainable, and ultimately, more profitable operations. This isn’t just about spreadsheets; it’s about harnessing the power of cutting-edge technology to create farms that are both productive and environmentally responsible.The next decade will see a dramatic increase in the sophistication and accessibility of data analytics tools for farmers.
This will be driven by advancements in computing power, the proliferation of low-cost sensors, and the increasingly user-friendly nature of data science platforms. Imagine a farm where every aspect, from soil moisture levels to individual plant health, is constantly monitored and analyzed, allowing for proactive adjustments and optimized resource allocation. This is the reality we’re rapidly approaching.
Artificial Intelligence and Machine Learning in Farm Management
AI and machine learning are no longer futuristic fantasies; they are actively reshaping the agricultural landscape. These technologies can analyze vast datasets to identify patterns and predict outcomes, providing farmers with invaluable insights for decision-making. For example, AI-powered systems can predict crop yields with unprecedented accuracy, optimize irrigation schedules to minimize water waste, and even detect diseases or pests before they cause significant damage.
Precision agriculture, driven by AI, will allow farmers to target resources precisely where they are needed, maximizing efficiency and minimizing environmental impact. Think of it as having a tireless, hyper-intelligent farmhand working 24/7, analyzing data and providing actionable recommendations. Companies like John Deere are already integrating AI into their machinery, offering farmers real-time feedback and automated adjustments.
Visual Representation of Future Impact
Imagine a vibrant infographic. The left side depicts a traditional farm, with somewhat haphazard resource allocation – water sprinklers showering dry patches and fertilizer spread unevenly. The yield is represented by a moderately sized bushel of crops. On the right, a futuristic farm is shown. This farm utilizes drones equipped with multispectral cameras, providing real-time data on crop health.
Sensors monitor soil conditions and water levels, automatically adjusting irrigation and fertilization. The yield is dramatically larger, a bursting, overflowing bushel, showcasing the increased efficiency and productivity. The background of the futuristic farm shows lush, healthy vegetation, contrasting with the slightly drier landscape of the traditional farm, visually representing the enhanced sustainability achieved through data-driven practices. A small bar graph next to the bushels visually compares the yield and profit margins between the two farming approaches, clearly showing the significant increase in both sustainability and profitability achieved through data analytics.
This visual representation effectively demonstrates the transformative potential of data analytics in enhancing both the economic viability and environmental responsibility of millennial farms.
Closing Summary
So, there you have it: the millennial farmer, a modern-day agricultural superhero armed with data. They’re proving that farming isn’t just about getting your hands dirty; it’s about getting smart with your data. By embracing technology and analytics, these farmers are not only boosting their efficiency and profitability but also paving the way for a more sustainable and innovative future in agriculture.
It’s a testament to the power of combining tradition with technology, resulting in a bountiful harvest of both crops and knowledge. The future of farming is digital, and it’s looking incredibly fertile.